- The Product-Led Geek
- Posts
- 👓 How to write a great experiment plan + PLGeek Notion Template
👓 How to write a great experiment plan + PLGeek Notion Template
Good morning folks! In today’s PLGeek:
📅 GEEKS OF THE WEEK: 5 links for you to bookmark
🧠 GEEK OUT: How to write a great experiment plan
📝 GEEK TEMPLATE: My go-to experiment plan template (Notion)
😂 GEEK GIGGLE: 1 thing that made me laugh this week.
Total reading time: 8 minutes
Let’s go!
Upload customer interviews, research papers, support tickets, NPS responses - all your qualitative data into Dovetail and use Magic search to get quick clarity on your next big decision.
Magic search can:
Understand the meaning behind questions
Retrieve supporting data
Summarize everything in a shareable video highlight reel
Get access to Magic search and all Dovetail’s AI features on our Professional plan. Available today for Product-Led Geek subscribers for an exclusive extended 30-day trial.
Please support our sponsors!
📅 GEEKS OF THE WEEK
5 bookmark-worthy links:
🧠 GEEK OUT
How to write a great experiment plan
Well-crafted experiment plan are more than just documents - they’re the foundation of successful testing programs.
They guide you through the process of testing hypotheses and driving meaningful improvements in your product.
A great experiment plan template sets a high bar for product and growth teams to uphold the growth process and minimises the risk of costly experiment planning and execution errors.
In this post, I’m going to share the experiment plan template that I’ve used and evolved over my time as a product and growth leader, including most recently at Snyk, as well as with many of the companies I’ve advised.
I’ll go through my template section by section and break it all down for you.

But before we get going, ask yourselves - “Do we really need an experiment for this?”
There are several reasons than an experiment might not make sense:
We have such high confidence in doing something that just moving forward with the implementation/scaling of an idea is low risk.
We don’t have enough traffic to this product surface area to make it feasible to run an experiment within a timeframe we feel is acceptable for learning
The questions we're trying to answer more 'why?' than 'what?'
We don’t have a well-informed and well-formed hypothesis.
For more on that take a look at the post below:
Assuming you determine an experiment is the right way forward…..Let’s dig in…
1. Summary & Learning Objective
Start with a clear, concise description of your experiment. Here's a simple format to follow:
"We want to [do this thing in the product] for [this group of users]. We hope to improve [this metric], and do no harm to [this metric]. When this experiment concludes, we hope to have learnt [learning objective]."
Why it's important: This section sets the stage for your entire experiment, providing a quick overview for stakeholders and team members. It's crucial because it:
Aligns everyone on the experiment's purpose and scope
Clearly states what you're trying to achieve and learn
Helps in quick decision-making by highlighting the key metrics you're focusing on
Sets expectations for the experiment's outcome
A well-defined summary and learning objective helps you stay focused throughout the experiment process and makes it easier to communicate your intentions to the wider team.
2. Hypothesis
Your hypothesis is the backbone of your experiment. It should be grounded in prior data, not just opinions. Use this format:
"Because [some insight/learnings/data/evidence we have], we believe that [doing this thing] will result in [some change to this metric]."
Why it's important: A strong hypothesis is critical because it:
Forces you to articulate your assumptions clearly
Helps people understand the ‘why’ behind the experiment
Ensures your experiment is based on evidence, not just hunches
Provides a clear prediction that you can test against
Helps you avoid running experiments without a clear purpose
Remember, great hypotheses are evidence-based. Link to research collections, analytics charts, or other supporting evidence. Think about your evidence in two parts:
The observation of the current situation (e.g. only 1.8% of users on this screen click the CTA), and
Why you think the test experience will change the observed situation (e.g. user research suggests that the CTA is not noticed, and a competing CTA that is more prominent is clicked by 16.4% of users)
In my experience, hypotheses that are grounded in solid evidence lead to accelerated learning.
Example:
Because only 1.8% of visitors to the homepage click on the CTA to sign up, and a competing CTA to view a demo is clicked by 16.4% of users, and recent user research suggests the signup CTA is not noticed,
We believe that changing the location of the signup CTA to make it more prominently visible,
Will result in more visitors seeing and interacting with the CTA.
3. Evidence
Elaborate on the evidence supporting your hypothesis. This might include analytics charts, session replays, user interview recordings, competitive analysis, or other market research.
Why it's important: The evidence section is crucial because it:
Validates the basis of your experiment
Provides context for your hypothesis
Helps others understand your reasoning
Can highlight potential areas of post experiment investigation to better understand the reasons behind a given outcome
The more comprehensive your evidence, the stronger your experiment foundation. I’ve seen time and time again that experiments with well-documented evidence are more likely to gain stakeholder buy-in and lead to meaningful product improvements.
4. Experience
This is the part where you describe how users will experience the experiment.
Clearly define both the control and test groups:
Control Group: Summarise what the control group will experience. Include screenshots/designs
Test Group: Detail the experience for the test/treatment group. Again, screens are a must.
Why it's important: Clearly defining the experience is critical because it:
Ensures everyone understands exactly what is being tested
Helps identify potential confounding variables
Provides a clear visual aid for implementation
Aids in interpreting the results by clearly showing what changed
Detailed descriptions of the control and test experiences help bring the test to life.
5. Targeting
Create a table outlining the following parameters:
Where: Which product surface will host the experiment?
Who: Which users will see the experiment?
When: When will those users see the experiment? To avoid bias in the experiment, this should be the very last moment before the user experience diverges for control and test group, i.e. when each set of users is bucketed in code.
How: How will traffic be split between test groups?
Why it's important: Precise targeting is crucial because it:
Ensures you're testing with the right audience
Helps control for variables that might skew your results
Allows for more accurate interpretation of results
Can highlight segment-specific insights
Well-defined targeting is essential to the integrity of your experiment.
6. Metrics
Clearly define the metrics that are relevant to your experiment.
Primary Metric: The main metric you're aiming to improve
Secondary Metrics: Other metrics you'll monitor
Guardrail Metric: A metric to ensure you're not negatively impacting crucial areas
For each, explain how it will be measured.
Why it's important: Well-defined metrics are essential to:
Provide clear success criteria for your experiment
Help you understand the full impact of your changes
Allow you to catch any unintended consequences
Enable informed decision making
Having a clear primary metric, along with secondary and guardrail metrics, helps you make more balanced decisions about implementing changes post-experiment.
7. Statistical Design
This section is crucial for ensuring your experiment's validity. Include:
Primary Metric Baseline
Target Runtime
Required Sample Size
Statistical Power
Significance Level
Minimum Detectable Effect (MDE)
Use a statistical design tool (example) to help determine these parameters.
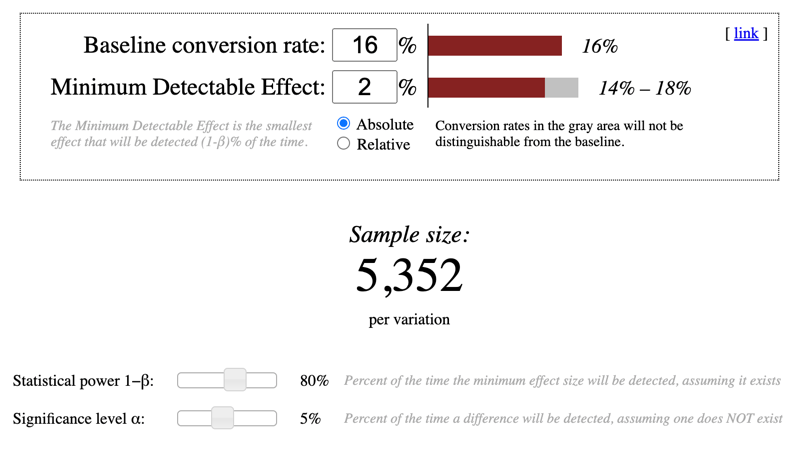
Evan Miller’s Sample Size Calculator
Why it's important: Robust statistical design is the foundation of any valid experiment. It
Helps you determine how long to run your experiment
Allows you to detect meaningful changes in your metrics
Ensures you know when your results are statistically significant and not due to chance
Increases wider confidence in your experiment results
If you don’t get this right, you’re very likely to be misled by data.
8. Action Plan
Outline your plan for various outcomes:
What constitutes success?
What's considered uncertain?
What's deemed a failure? (Still a learning?)
How will you analyse and split the data?
Who might find the experiment results interesting or useful?
Why it's important: A clear action plan is vital because it:
Prepares you for all possible outcomes
Speeds up decision-making post-experiment
Ensures you've thought through the implications of your results
Helps align stakeholders on next steps before you even start
Having a predetermined action plan helps you move quickly from experiment results to implementation, significantly improving your product iteration speed.
9. Results
After running your experiment, document the outcome:
What were the results?
Which variant won and why?
What was the impact on secondary and guardrail metrics?
Did you meet your learning objective?
What are the next steps?
Are any follow-ups needed?
Link to charts or notebooks that document the experiment data.
Why it's important: Thorough documentation of results is crucial because it:
Creates a record of what was learned
Helps inform future experiments and product decisions
Allows for knowledge sharing across the organisation
Provides accountability for the experiment process
Well-documented experiment results become a valuable resource, informing product and growth strategy and helping you avoid repeating unsuccessful experiments.
Bringing it all together
By following this structure and understanding the importance of each section, you'll create comprehensive, actionable experiment plans where each component plays an important role in ensuring your experiments are well-designed, executed, and leveraged for maximum impact.
Remember, the key to successful experimentation is rigour and clarity. Each section should be well-thought-out and clearly communicated. This not only helps your team execute the experiment effectively but also makes it easier to learn from the results and apply those learnings to future experiments.
In my time at Snyk, well-documented experiments were instrumental to our process of driving improvements to our growth metrics. They allowed us to make better decisions and continuously improve our product experience. As an additional benefit, they created a culture of experimentation where team members felt empowered to test their ideas and contribute to the product's growth.
Get this foundational discipline right and you’re half way there.
PS: Get the full Notion template below - feel free to duplicate it and use as you wish!
📝 GEEK TEMPLATE
Click here or on the image above to get the template.
😂 GEEK GIGGLE
Bootstrapped founder pitching VCs
— Enzo Avigo (@0zne)
8:00 PM • Aug 4, 2024
💪 POWER UP
If you enjoyed this post, consider upgrading to a VIG Membership to get the full Product-Led Geek experience and access to every post in the archive including all guides. 🧠
THAT’S A WRAP
Before you go, here are 2 ways I can help:
Book a free 1:1 consultation call with me - I keep a handful of slots open each week for founders and product growth leaders to explore working together and get some free advice along the way. Book a call.
Sponsor this newsletter - Reach over 7500 founders, leaders and operators working in product and growth at some of the world’s best tech companies including Paypal, Adobe, Canva, Miro, Amplitude, Google, Meta, Tailscale, Twilio and Salesforce.

That’s all for today,
If there are any product, growth or leadership topics that you’d like me to write about, just hit reply to this email or leave a comment and let me know!
Until next time!
— Ben

Rate this post (one click) |
PS: Thanks again to our sponsor: Dovetail
Reply